Loyal wingmen and Artificial Intelligence Development : a state of artDrones are increasingly present in everyday life. Their use has also increased in the fields of security and defense, where they take on the 5D task (Dull, Dirty, Dangerous, Dear and Di
Keywords:
Drones, Loyal Wingmen, Artificial Intelligence (AI)Abstract
Drones are increasingly present in everyday life. Their use has also increased in the fields of security and defense, where they take on the 5D task (Dull, Dirty, Dangerous, Dear and Difficult) and help protect men in the performance of risky tasks, as well as enhance the visibility and surveillance of disturbed and/or extended areas. The evolution of drones means the rise of artificial intelligence, especially for the most sophisticated ones, such as Loyal Wingmen. With the Russian invasion of Ukraine, drones are the focus of particular interest, and the US Department of Defense (DoD) place an order for 1,000 collaborative fighter jets by Loyal Wingmen is in the spotlight. In this context, this article will present the main challenges that AI must meet to allow the development of this type of specific drones and will make a state of the art of the development of Loyal Wingmen. Finally, in the light of the issues identified, this paper will propose an industrial development path.
Downloads
References
Altmann, J. & Sauer, F. 2017. Autonomous Weapon Systems and Strategic Stability Survival, Vol. 59, No. 5, pp. 117–142, https://doi.org/10.1080/00396338.2017.1375263.
al-Garni, A. D. 2022. Drones in the Ukrainian war : will they be an effective weapon in future war? Rasanah study
Arrieta, A. B., Díaz-Rodríguez, N., Del Ser, J., Bennetot, A., Tabik, S., Barbado, A., ... & Herrera, F. (2020). Explainable Artificial Intelligence (XAI): Concepts, taxonomies, opportunities and challenges toward responsible AI. Information fusion, 58, 82-115.
Brumfield, E. 2014. Armed Drones for Law Enforcement: Why It Might Be Time to Re-Examine the Current Use of Force Standard. McGeorge Law Rev, 46, 543
Bryen, S. 2017. Armed Drones Revolutionizing the Future of War, Asia Times, December 9, 2020, https://asiatimes.com/2020/12/armed-drones-revolutionizing-the-future-of-war/.
Calcara, A., Gilli,A., Gilli,M., Marchetti,R., Zaccagnini, I. 2022. Why Drones Have Not Revolutionized War: The Enduring Hider-Finder Competition in Air Warfare. International Security 2022; 46 (4): 130–171. doi: https://doi.org/10.1162/isec_a_00431
Cansell,P. & Desmoulin, L. 2017. De la RMA à la guerre infocentrée : retours d’expérience sur les promesses inaccomplies de la numérisation et du big data. COSSI - Méthodes et stratégies de gestion de l’information par les organisations : des ”big data” aux ”thick data, May 2017, Montréal, France. p.50-61. .hal-02984391.
Chávez, K. (2023). Learning on the Fly: Drones in the Russian-Ukrainian War. Arms Control Today, 53(1), 6-11.
Chen, M., Li, B., Wang, J., & Wang, G. (2022, November). A Formation Control Algorithm for Air-Ground Cooperative UAV. In 2022 14th International Conference on Wireless Communications and Signal Processing (WCSP) (pp. 741-747). IEEE.
Davis, N. C. (1997). An information-based revolution in military affairs. RAND-PUBLICATIONS-MR-ALL SERIES-, 79-98.
de Henning Michaëlis, S. (2023). Turkey’s And Iran’s Drone Supply In The War In Ukraine. Policy, (21).
Duan, HB., Ma, GJ. & Luo, DL. 2008. Optimal formation reconfiguration control of multiple UCAVs using improved particle swarm optimization. Journalof Bionic Engineering, 5(4):340-347 doi:10.1016/S1672-6529(08)60179-1.
Duan, HB. & Liu, SQ. 2010. Non-linear dual-mode receding horizon control for multiple
unmanned air vehicles formation flight based on chaotic particle swarm optimisation. Control Theory & Applications, IET, 4(11):2565{2578, 2010.
doi:10.1049/iet-cta.2009.0256.
Dugoin-Clément. C. 2023. Ukraine-Russie : un an de guerre des UAVs. DSI
Dugoin-Clément, C. 2023. Ukraine, Russie et high-tech, un an après le déclenchement de l’invasion. Revue Défense Nationale, (2), 37-42.
Dwivedi, R., Dave, D., Naik, H., Singhal, S., Omer, R., Patel, P., ... & Ranjan, R. (2023). Explainable AI (XAI): Core ideas, techniques, and solutions. ACM Computing Surveys, 55(9), 1-33.
Eslami, M. 2022. Iran’s drone supply to Russia and changing dynamics of the Ukraine war. Journal for Peace and Nuclear Disarmament, 5(2), 507-518.
Fuhrmann, M. & Horowitz, M.C. 2017. “Droning On: Explaining the Proliferation of Unmanned Aerial Vehicles,” International Organization, Vol. 71, No. 2, 397–418, https://doi.org/10.1017/S0020818317000121.
Furukawa,T., Durrant-Whyte, HF., Bourgault, F. & Dissanayake, G. 2003. Time-optimal coordinated control of the relative formation of multiple vehicles. In Computational Intelligence in Robotics and Automation, Proceedings 2003 IEEE International Symposium on, volume 1, 259-264. IEEE, 2003. DOI: 10.1109/CIRA.2003.1222099.
Garcia, RD., Barnes, L & Fields, MA. 2012. Unmanned aircraft systems as wingmen. The Journal of Defense Modeling and Simulation: Applications, Methodology, Technology, 9(1):5{15, 2012. doi:10.1177/1548512910391217.
Giacomossi, L., Schwanz Dias, S., Brancalion J. F. and Maximo, M. R. O. A. 2021 "Cooperative and Decentralized Decision-Making for Loyal Wingman UAVs," 2021 Latin American Robotics Symposium (LARS), 2021 Brazilian Symposium on Robotics (SBR), and 2021 Workshop on Robotics in Education (WRE), Natal, Brazil, 2021, pp. 78-83, doi: 10.1109/LARS/SBR/WRE54079.2021.9605468.
Giulietti,F., Pollini, L & Innocenti, M. 2000. Autonomous formation flight. Control Systems, IEEE, 20(6):34{44, 2000.
Gunning, D., Stefik, M., Choi, J., Miller, T., Stumpf, S., & Yang, G. Z. (2019). XAI—Explainable artificial intelligence. Science robotics, 4(37), eaay7120.
Gunning, D., & Aha, D. (2019). DARPA’s explainable artificial intelligence (XAI) program. AI magazine, 40(2), 44-58.
Harl , N. and Balakrishnan, SN. 2008. Coordinated rendezvous of unmanned air vehicles
to a formation: A sliding mode approach. In AIAA Guidance, Navigation and Control Conference, 2008.
Hecht, E. (2022). Drones in the Nagorno-Karabakh War: Analyzing the Data. Mil. Strateg. Mag, 7, 31-37.
Houck, M. R., Whitaker L. A. and Kendall, R. R. 1992 "A cognitive classification of pilot performance in air combat," Proceedings of the IEEE 1992 National Aerospace and Electronics Conference@m_NAECON 1992, Dayton, OH, USA, 1992, pp. 503-509 vol.2, doi: 10.1109/NAECON.1992.220524.
Hu, X. & Eberhart, R. 2002. Solving constrained nonlinear optimization problems with particle swarm optimization. In Proceedings of the Sixth World Multiconference on Systemics, Cybernetics and Informatics, volume 5, pages 203-206. Citeseer, 2002.
Humphreys, C. J. (2016). Optimal control of an uninhabited loyal wingman.
Kinik, H., & Çelik, S. (2021). The Role of Turkish Drones in Azerbaijan’s Increasing Military Effectiveness. Insight Turkey, 23(4), 169-192.
Kunertova, D. 2023. The war in Ukraine shows the game-changing effect of drones depends on the game. Bulletin of the Atomic Scientists, 79(2), 95-102
Kovacina MA., Palmer, D., Yang, G. & Vaidyanathan, R. 2002. Multi-agent control algorithms for chemical cloud detection and mapping using unmanned air vehicles. In International Conference on Intelligent Robots and Systems, volume 3, 2782-2788. IEEE, 2002. doi:10.1109/IRDS.2002.1041691.
Li, B., Zhang, J., Dai, L., Teo, K. L., & Wang, S. (2020). A hybrid offline optimization method for reconfiguration of multi-UAV formations. IEEE Transactions on Aerospace and Electronic Systems, 57(1), 506-520.
Ling, KV., Maciejowski,J., Richards,A. & Wu, BF. 2012. Multiplexed model predictive control. Automatica, 48(2):396-401.
Liu, G., Li, B., & Ji, Y. (2022). A modified HP-adaptive pseudospectral method for multi-UAV formation reconfiguration. ISA transactions, 129, 217-229.
Losing, S. 2023. US Air Force eyes fleet of 1,000 drone wingmen as planning accelerates
Defense News, March 8th
Ma, G., Huang, H. & Zhuang, Y. 2010. Time optimal trajectory planning for reconfiguration of satellite formation with collision avoidance. In Control and Automation (ICCA), 2010 8th IEEE International Conference on, 476-479. IEEE, 2010.
Mahadevan, P. 2010. The military utility of drones. CSS Analyses in Security Policy, 78.
Marin, L.; Krajčíková, K. 2016. Deploying Drones in policing southern European borders: Constraints and challenges for data protection and human rights. In Drones and Unmanned Aerial Systems; Springer: Berlin/Heidelberg, Germany, pp. 101–127
McDermott, R. 2021. Moscow Forming First Robotic Military Units, Eurasia Daily Monitor Volume: 18 Issue: 64
Meske, C., Bunde, E., Schneider, J., & Gersch, M. (2022). Explainable artificial intelligence: objectives, stakeholders, and future research opportunities. Information Systems Management, 39(1), 53-63.
Mohanavelu, K., Poonguzhali, S., Adalarasu, K., Ravi, D., Chinnadurai, V., Vinutha, S., ... & Jayaraman, S. (2020). Dynamic cognitive workload assessment for fighter pilots in simulated fighter aircraft environment using EEG. Biomedical Signal Processing and Control, 61, 102018.
Park, S. 2003. Avionics and Control System Development for Mid-Air Rendezvous of Two Unmanned Aerial Vehicles. PhD thesis, Massachusetts Institute of Technology, 2003.
Ree, M. J., & Carretta, T. R. (1996). Central role of g in military pilot selection. The International Journal of Aviation Psychology, 6(2), 111-123.
Roma, A. 2017. Drones and popularisation of space, Space Policy, 41, 65–67
Russell, MA. & Lamont, GB. 2005. A genetic algorithm for unmanned aerial vehicle routing. In Proceedings of the 7th Annual Conference on Genetic and Evolutionary Computation, 1523-1530. ACM.
Sammut, C & Webb, GI. 2011. Encyclopedia of Machine Learning. Springer Science & Business Media.
Shishkov, B.; Hristozov, S.; Janssen, M.; van den Hoven, J. 2017. Drones in Land Border Missions: Benefits and Accountability Concerns. In Proceedings of the 6th International Conference on Telecommunications and Remote Sensing, Delft, The Netherlands, 6–7 November 2017; p. 77–86.
Siau, K & Wang W. 2020. Building Trust in Artificial Intelligence, Machine Learning, and Robotics, Cutter Business Technology Journal, Vol. 31, No. 2
Sloan, E. C. 2002. Revolution in Military Affairs (Vol. 5). McGill-Queen's Press-MQUP
Smith.AL. 2008. Proportional navigation with adaptive terminal guidance for aircraft rendezvous. Journal of Guidance, Control, and Dynamics, 31(6):1832{1836, 2008. doi: 10.2514/1.33535.
Stelmack, K. 2014. Weaponized Police Drones and Their Effect on Police Use and Force. J. Technol. Law Policy, 15, 276
Stickney., HM 2014. Performance characterization, development, and application
of artifcial potential function guidance methods. Technical report, DTIC Document, 2014.
Stulberg, AN. 2017. Managing the Unmanned Revolution in the U.S. Air Force,” Orbis, Vol. 51, No. 2 (2007), pp. 251–265, https://doi.org/10.1016/j.orbis.2007.01.005
Tahk, MJ., Park,CS and Ryoo. CK. 2005. Line-of-sight guidance
laws for formation flight. Journal of Guidance, Control, and Dynamics, 28(4):708{716, 2005. doi: 10.2514/1.9605.
Tanner, HG., Jadbabaie,A and Pappas, GJ. 2003 Stable flocking of mobile agents, part i: Fixed topology. In Decision and Control, Proceedings of the 42nd IEEE Conference on, volume 2, pages 2010{2015. IEEE, 2003.
Teodorovic , D & Dell’Orco, M. 2005. Bee colony optimization{a cooperative learning approach to complex transportation problems. In Advanced OR and AI Methods in Transportation, Proceedings of 16th Mini EURO Conference, 51-60. Poznan Publishing House of the Polish Operational and System Research.
Tikanmäki, I.2011. Possibilities to Operational Use of Remotely Piloted Aircrafts in Finland. Master’s Thesis, Laurea-ammattikorkeakoulu, Vantaa, Finland, 2011.
Tjoa, E., & Guan, C. (2020). A survey on explainable artificial intelligence (xai): Toward medical xai. IEEE transactions on neural networks and learning systems, 32(11), 4793-4813.
Tsiamis, N., Efthymiou, L., & Tsagarakis, K. P. 2019. A comparative analysis of the legislation evolution for drone use in OECD countries. Drones, 3(4), 75.
Villasenor, J. 2014. “Drones” and the future of domestic aviation. Proc. IEEE, 102, 235–238
Wang, X., Yadav, V., & Balakrishnan, S. N. (2007). Cooperative UAV formation flying with obstacle/collision avoidance. IEEE Transactions on control systems technology, 15(4), 672-679.
Weihua, Z. &Go, TH.2011. Robust decentralized formation fliight control. International Journal of Aerospace Engineering.
Witt, S. 2023. The Turkish Drone That Changed the Nature of Warfare, the NewYorker, May 16th, 2022.
Xie, Y., Han, L., Dong, X., Li, Q., & Ren, Z. (2021). Bio-inspired adaptive formation tracking control for swarm systems with application to UAV swarm systems. Neurocomputing, 453, 272-285.
Zhang, B., Sun, X., Liu, S., & Deng, X. (2019). Adaptive differential evolution-based receding horizon control design for multi-UAV formation reconfiguration. International Journal of Control, Automation and Systems, 17(12), 3009-3020.
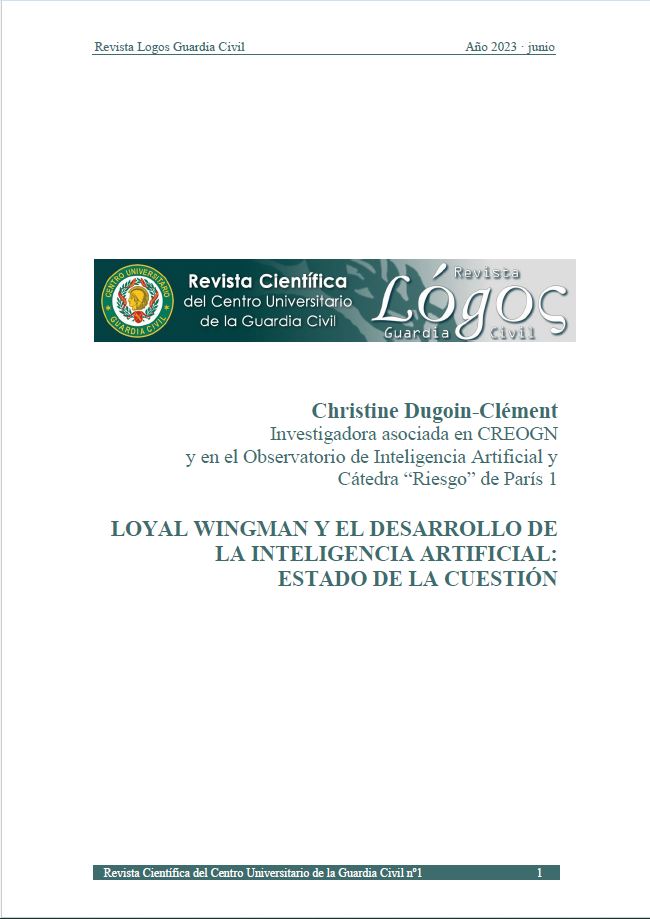
Downloads
Published
How to Cite
Issue
Section
License
Copyright (c) 2023 Christine Dugoin-Clément

This work is licensed under a Creative Commons Attribution-NonCommercial-NoDerivatives 4.0 International License.
-
Attribution — You must give appropriate credit, provide a link to the license, and indicate if changes were made. You may do so in any reasonable manner, but not in any way that suggests the licensor endorses you or your use.
-
NonCommercial — You may not use the material for commercial purposes.
-
NoDerivatives — If you remix, transform, or build upon the material, you may not distribute the modified material.
- No additional restrictions — You may not apply legal terms or technological measures that legally restrict others from doing anything the license permits.