SISTEMA AVANZADO DE AYUDA A LA CONDUCCIÓN (ADAS) EN ROTONDAS /GLORIETAS USANDO IMÁGENES AÉREAS Y TÉCNICAS DE INTELIGENCIA ARTIFICIAL PARA LA MEJORA DE LA SEGURIDAD VIAL
Keywords:
Roundabouts, Machine Learning, Aerial Imagery, Object Detection, Deep Learning, Computer VisionAbstract
Roundabouts are a type of traffic construction in which several roads converge and communicate through a rotating circulation around a central island. Although, they have increased safety, driving around them properly is not an easy task for conventional and autonomous vehicles. There are publications about ADAS that considers roundabouts as objects to be transited, guiding these autonomous ones in the circulation. This work present takes roundabouts as a source of information that could be transmitted to these vehicles to improve their decision making. To this end, it details the creation of a prototype for monitoring Spanish roundabouts using aerial imagery and machine learning. This system requires an installation phase in which it is calibrated using image processing techniques to recognize the circumferences of the central island and the different lanes. Then, using a RetinaNET model based on a resnet50 backbone, each vehicle and its type is detected. Combining both subsystems, the prototype extract information about the position of vehicles (exact location and lane), entering/exiting ones and those that affect the entering (key aspect for capacity calculation). The aim is to improve security by using artificial intelligence techniques.
Downloads
References
Akçelik, R. (2004). Roundabouts with unbalanced flow patterns. Paper presented at the Institute of Trans- portation Engineers 2004 Annual Meeting.
Anagnostopoulos, A., Kehagia, F., Damaskou, E., Mouratidis, A., & Aretoulis, G. (2021). Predicting Roundabout Lane Capacity using Artificial Neural Networks. Journal of Engineering Science and Technology Review, 14, 210-215.
Badue, C., Guidolini, R., Carneiro, R., Azevedo, P., Cardoso, V., Forechi, A., . . . De Souza, A. (2019). Self-Driving Cars: A Survey,.
Ballard, D., & Brown, C. (1982). Computer vision. englewood cliffs. J: Prentice Hall.
BBC. (2020, 09 16). Uber's self-driving operator charged over fatal crash. Retrieved 12 31, 2021, from https://www.bbc.com/news/technology-54175359
BBC. (2021, 12 15). Tesla Model 3: Paris' largest taxi firm suspends cars after fatal crash. Retrieved 12 31, 2021, from https://www.bbc.com/news/world-europe-59647069
BCG. (2015). A Roadmap to Safer Driving through Advanced Driver Assistance Systems. Retrieved 12 30, 2021, from https://image-src.bcg.com/Images/MEMA-BCG-A-Roadmap-to-Safer-Driving-Sep-2015_tcm9-63787.pdf
Behrisch, M., Bieker, L., Erdmann, J., & Krajzewicz, D. (2011). SUMO - Simulation of Urban MObility.
Bernhard, W., & Portmann, P. (2000). Traffic simulation of roundabouts in Switzerland. 2000 Winter Simulation Conference Proceedings (Cat. No.00CH37165), 2, 1148-1153.
Bochkovskiy, A., Wang, C.-Y., & Liao, H.-Y. (2020). Bochkovskiy, Alexey, Chien-Yao Wang, and Hong-Yuan Mark Liao. "Yolov4: Optimal speed and accuracy of object detection. arXiv preprint arXiv:2004.10934.
Bock, J., Krajewski, R., Moers, T., & Vater, L. (n.d.). UniD. The University Drone Dataset. Naturalistic Trajectories of Vehicles and Vulnerable Road Users Recorded at the RWTH Aachen University Campus. Retrieved 03 26, 2022, from https://www.unid-dataset.com/
Bock, J., Krajewski, R., Moers, T., Runde, S., Vater, L., & Eckstein, L. (2020). The inD Dataset: A Drone Dataset of Naturalistic Road User Trajectories at German Intersections. 2020 IEEE Intelligent Vehicles Symposium (IV), 1929-1934.
Breuer, A., Termöhlen, J.-A., Homoceanu, S., & Fingscheidt, T. (2020). OpenDD: A Large-Scale Roundabout Drone Dataset. Proceedings of International Conference on Intelligent Transportation Systems}.
Brilon, W. (1991). Intersections Without Traffic Signals II: Proceedings of an International Workshop. Springer-Verlag.
Brilon, W., Wu, N., & Bondzio, L. (1997). Unsignalized intersections in Germany — a state of the art 1997. Third international symposium on intersections without traffic signals, 61–70.
Canny, J. (1986). A Computational Approach to Edge Detection. IEEE Transactions on Pattern Analysis and Machine Intelligence, PAMI-8(6), 679-698.
Chang, Y.-C., Huang, C., Chuang, J.-H., & Liao, I.-C. (2018). Pedestrian Detection in Aerial Images Using Vanishing Point Transformation and Deep Learning. 2018 25th IEEE International Conference on Image Processing (ICIP), 1917-1921.
Chen, Z., Wang, C., Wen, C., Teng, X., Chen, Y., Guan, H., . . . Li, J. (2015). Vehicle detection in high-resolution aerial images via sparse representation and superpixels. IEEE Transactions on Geoscience and Remote Sensing, 54(1), 103-116.
Cheng, G., Wang, Y., Xu, S., Wang, H., Xiang, S., & Pan, C. (2017). Automatic Road Detection and Centerline Extraction via Cascaded End-to-End Convolutional Neural Network. IEEE Transactions on Geoscience and Remote Sensing, 55(5), 3322-3337.
Chin, H. C. (1985). SIMRO: A model to simulate traffic at roundabouts. Traffic Engineering and Control, 26, 109–113.
Chung, E., Young, W., & Akçelik, R. (1992). Comparison of roundabout capacity and delay estimates from analytical and simulation models. 16th ARRB Conference, 16, 369–385.
Dalal, N., & Triggs, B. (2005). Histograms of Oriented Gradients for Human Detection. In Proceedings of the 2005 IEEE Computer Society Conference on Computer Vision and Pattern Recognition, 1, 886-893.
De-Las-Heras, G., Sánchez-Soriano, J., & Puertas, E. (2021). Advanced Driver Assistance Systems (ADAS) Based on Machine Learning Techniques for the Detection and Transcription of Variable Message Signs on Roads. Sensors, 21(17).
Deng, Z., Sun, H., Zhou, S., Zhao, J., & Zou, H. (2017). Toward fast and accurate vehicle detection in aerial images using coupled region-based convolutional neural networks. IEEE Journal of Selected Topics in Applied Earth Observations and Remote Sensing, 10(8), 3652--3664.
Dijkstra, K., van de Loosdrecht, J., Schomaker, L. R., & Wiering, M. A. (2019). Hyperspectral demosaicking and crosstalk correction using deep learning. Machine Vision and Applications, 30, 1-21.
Dirección General de Tráfico - Ministerio del Interior. (2021, 12 23). Código de Tráfico y Seguridad Vial. Retrieved 01 01, 2022, from https://www.boe.es/biblioteca_juridica/codigos/abrir_pdf.php?fich=020_Codigo_de_Trafico_y_Seguridad_Vial.pdf
Dirección General de Tráfico - Ministerio del Interior. (2018). Cuestiones De Seguridad Vial. Retrieved 12 30, 2021, from http://www.dgt.es/Galerias/seguridad-vial/formacion-vial/cursos-para-profesores-y-directores-de-autoescuelas/XXI-Cuso-Profesores/Manual-II-Cuestiones-de-Seguridad-Vial-2018.pdf
DJI. (n.d.). DJI Mini 2. Retrieved 03 26, 2022, from https://www.dji.com/ca/mini-2/specs
Du, D., Qi, Y., Yu, H., Yang, Y., Duan, K., Li, G., . . . Tian, Q. (2018). The Unmanned Aerial Vehicle Benchmark: Object Detection and Tracking. European Conference on Computer Vision (ECCV).
Elkhrachy, I. (2021). Accuracy Assessment of Low-Cost Unmanned Aerial Vehicle (UAV) Photogrammetry. Alexandria Engineering Journal, 60(6), 5579-5590.
Everingham, M., Van Gool, L., & Williams, C. (2010). The Pascal Visual Object Classes (VOC) Challenge. International Journal of Computer Vision, 88, 303-338.
Felzenszwalb, P., McAllester, D., & Ramanan, D. (2008). A discriminatively trained, multiscale, deformable part mode, computer vision and pattern recognition. 2008 IEEE Conference on Computer Vision and Pattern Recognition, 1-8.
fizyr. (n.d.). Keras RetinaNet. Retrieved 04 05, 2022, from https://github.com/fizyr/keras-retinanet
Gallardo, V. (2005). Funciones de las rotondas urbanas y requerimientos urbanísticos de organización.
García Cuenca, L., Puertas, E., Fernandez Andrés, J., & Aliane, N. (2019). Autonomous Driving in Roundabout Maneuvers Using Reinforcement Learning with Q-Learning. Electronics, 8(12).
García Cuenca, L., Sanchez-Soriano, J., Puertas, E., Fernandez Andrés, J., & Aliane, N. (2019). Machine Learning Techniques for Undertaking Roundabouts in Autonomous Driving. Sensors, 19(10).
Girshick, R. (2015). Fast R-CNN. 2015 IEEE International Conference on Computer Vision (ICCV), 1440-1448.
Girshick, R., Donahue, J., Darrell, T., & Malik, J. (2013). Rich Feature Hierarchies for Accurate Object Detection and Semantic Segmentation. Proceedings of the IEEE Computer Society Conference on Computer Vision and Pattern Recognition.
Gonzalez, R., Woods, R., & Masters, B. (2009). Digital image processing.
Guichet, B. (1997). Roundabouts in France: Development, safety, design, and capacity. Proceedings of the third international symposium on intersections without traffic signals, 100–105.
Gupta, A., Watson, S., & Yin, H. (2021). Deep learning-based aerial image segmentation with open data for disaster impact assessment. Neurocomputing, 439, 22-33.
Hagring, O. (2000). Effects of OD Flows on Roundabout Entry Capacity. Fourth international symposium on highway capacity(434–445).
Hough, P. (1962, 12 18). U.S. Patent No. US3069654A.
ImageJ. (n.d.). Hough Circle Transform. Retrieved 01 16, 2022, from https://imagej.net/plugins/hough-circle-transform
Kathuria, A. (n.d.). Data Augmentation for Bounding Boxes: Rotation and Shearing. Retrieved 04 05, 2022, from https://blog.paperspace.com/data-augmentation-for-object-detection-rotation-and-shearing/
Kembhavi, A., Harwood, D., & Davis, L. S. (2010). Vehicle detection using partial least squares. IEEE Transactions on Pattern Analysis and Machine Intelligence, 33(6), 1250-1265.
Kim, J., Candemir, S., Chew, E. Y., & Thoma, G. R. (2018). Region of Interest Detection in Fundus Images Using Deep Learning and Blood Vessel Information. 2018 IEEE 31st International Symposium on Computer-Based Medical Systems (CBMS), 357-362.
Kimber, R. M. (1980). LR942 The traffic capacity of roundabouts. Transport and Road Research Laboratory.
Krajewski, R., Bock, J., Kloeker, L., & Eckstein, L. (2018). The highD Dataset: A Drone Dataset of Naturalistic Vehicle Trajectories on German Highways for Validation of Highly Automated Driving Systems. 2018 21st International Conference on Intelligent Transportation Systems (ITSC), 2118-2125.
Krajewski, R., Bock, J., Moers, T., & Vater, L. (n.d.). ExiD. The Exits and Entries Drone Dataset. Naturalistic Trajectories of Vehicles Recorded at Exits and Entries of German Highways . Retrieved 03 26, 2022, from https://www.exid-dataset.com/
Krajewski, R., Moers, T., Bock, J., Vater, L., & Eckstein, L. (2020). The rounD Dataset: A Drone Dataset of Road User Trajectories at Roundabouts in Germany. 2020 IEEE 23rd International Conference on Intelligent Transportation Systems (ITSC), 1-6, 2020.
Krizhevsky, A., Sutskever, I., & Hinton, G. E. (2017). ImageNet Classification with Deep Convolutional Neural Networks. Commun. ACM, 60(6), 84–90.
Kukkala, V. K., Tunnell, J., Pasricha, S., & Bradley, T. (2018). Advanced Driver- Assistance Systems: A Path Toward Autonomous Vehicles. IEEE Consumer Electronics Magazine, 18-25.
Lin, T.-Y., Goyal, P., Girshick, R., He, K., & Dollár, P. (2020). Focal loss for dense object detection. IEEE Transactions on Pattern Analysis and Machine Intelligence, 42(2), 318-327.
Liu, K., & Mattyus, G. (2015). Fast Multiclass Vehicle Detection on Aerial Images. IEEE Geoscience and Remote Sensing Letters, 12(9), 1938-1942.
Liu, W., Anguelov, D., Erhan, D., Szegedy, C., Reed, S., Fu, C.-Y., & Berg, A. (2016). Ssd: Single shot multibox detector. European conference on computer vision.
Macioszek, E. (2020). Roundabout Entry Capacity Calculation—A Case Study Based on Roundabouts in Tokyo, Japan, and Tokyo Surroundings. Sustainability, 12, 1533.
Marlow, M., & Maycock, G. (1982). SR724 The effect of Zebra crossings on junction entry capacities. Crowthorne: Transport and Road Research Laboratory.
Mauro, R., & Guerrieri, M. (2012). Right-turn Bypass Lanes at Roundabouts: Geometric Schemes and Functional Analysis. Modern Applied Science, 7.
Michon, J. (1993). Generic Intelligent Driver Support: A Comprehensive Report on GIDS.
Microsimulation, P. (2011). S-Paramics (Parallel Microscopic Traffic Simulator).
Montgomery, F., Tenekeci, G., & Wainaina, S. (2010). Roundabout capacity in adverse weather and light conditions. Proceedings of The Institution of Civil Engineers-transport - PROC INST CIVIL ENG-TRANSPORT, 163, 29-39.
Naranjo, J., Sotelo, M.-A., Gonzalez, C., Garcia, R., & Pedro, T. (2007). Using Fuzzy Logic in Automated Vehicle Control. Intelligent Systems, IEEE, 22, 36-45.
O'Kane, S. (2018, 04 19). How Tesla and Waymo are tackling a major problem for self- driving cars: data. Retrieved 01 01, 2022, from https://www.theverge.com/transportation/2018/4/19/17204044/tesla-waymo- self-driving-car-data-simulation
OpenCV. (n.d.). Canny Edge Detector . Retrieved 01 16, 2022, from https://docs.opencv.org/4.x/da/d5c/tutorial_canny_detector.html
OpenCV. (n.d.). Image Thresholding . Retrieved 01 15, 2022, from https://docs.opencv.org/4.x/d7/d4d/tutorial_py_thresholding.html
OpenCV. (n.d.). Morphological Transformations. Retrieved 01 15, 2022, from https://docs.opencv.org/3.4/d9/d61/tutorial_py_morphological_ops.html
OpenCV. (n.d.). Operations on arrays. Retrieved 01 15, 2022, from https://docs.opencv.org/3.4/d2/de8/group__core__array.html#ga6fef31bc8c4071cbc114a758a2b79c14
OpenCV. (n.d.). Smoothing Images. Retrieved 01 15, 2022, from https://docs.opencv.org/4.x/d4/d13/tutorial_py_filtering.html
OpenCV. Open Source Computer Vision. (n.d.). Hough Circle Transform . Retrieved 01 16, 2022, from https://docs.opencv.org/3.4/d4/d70/tutorial_hough_circle.html
OpenCV. Open Source Computer Vision. (n.d.). Hough Line Transform . Retrieved 01 16, 2022, from https://docs.opencv.org/4.x/d9/db0/tutorial_hough_lines.html
Popular Science. (2021, 12 30). Tesla caps off a tumultuous year with a massive recall. Retrieved 12 31, 2021, from https://www.popsci.com/technology/tesla-recalls-vehicles-safety-issues/
Puertas, E., De-Las-Heras, G., Fernández-Andrés, J., & Sánchez-Soriano, J. (2022). Dataset: Roundabout Aerial Images for Vehicle Detection. Data, 7(4).
Rebaza, J. (2007). Detección de Bordes Mediante el Algoritmo de Canny. Master’s Thesis. Trujillo, Peru: Universidad Nacional de Trujillo.
Redmon, J., & Farhadi, A. (2017). YOLO9000: Better, faster, stronger. Proceedings of the IEEE conference on computer vision and pattern recognition.
Redmon, J., & Farhadi, A. (2018). YOLOv3: An incremental improvement. arXiv preprint arXiv:1804.02767.
Redmon, J., Divvala, S., Girshick, R., & Farhadi, A. (2016). You only look once: Unified, real-time object detection. Proceedings of the 2016 IEEE Conference on Computer Vision and Pattern Recognition, 779–788.
Ren, S., He, K., Girshick, R., & Sun, J. (2017). Faster R-CNN: Towards real-time object detection with region proposal networks. IEEE Transactions on Pattern Analysis and Machine Intelligence, 39(6), 1137-1149.
Robicquet, A., Sadeghian, A., Alahi, A., & Savarese, S. (2016). Learning Social Etiquette: Human Trajectory Prediction In Crowded Scenes. European Conference on Computer Vision (ECCV).
Rodrigues, M., Gest, G., McGordon, A., & Marco, J. (2017). Adaptive behaviour selection for autonomous vehicle through naturalistic speed planning. 2017 IEEE 20th International Conference on Intelligent Transportation Systems (ITSC), 1-7.
Rodríguez, J. I. (2014). Cómo circular por una glorieta. Tráfico y seguridad Vial, Septiembre-Octubre(228), 28-30.
Rosebrock, A. (2015). Zero-Parameter, Automatic Canny Edge Detection with Python and OpenCV. Retrieved 01 16, 2022, from https://www.pyimagesearch.com/2015/04/06/zero-parameter-automatic-canny-edge-detection-with-python-and-opencv/
scikit-image development team. (n.d.). Circular and Elliptical Hough Transforms. Retrieved 01 16, 2022, from https://scikit-image.org/docs/stable/auto_examples/edges/plot_circular_elliptical_hough_transform.html
Sermanet, P., Eigen, D., Zhang, X., Mathieu, M., Fergus, R., & Lecun, Y. (2013). Over Feat: Integrated Recognition, Localization and Detection using Convolutional Networks. arXiv preprint arXiv:1312.6229.
Shehata, A., Mohammad, S., Abdallah, M., & Ragab, M. (n.d.). A survey on Hough transform, theory, techniques and applications. arXiv 2015, arXiv:1502.02160.
Shen, J., Liu, N., & Sun, H. (2021). Vehicle detection in aerial images based on lightweight deep convolutional network. IET Image Processing, 15(2), 479-491.
Silva, A., Vasconcelos, A., & Santos, S. (2014). Moving from Conventional Roundabouts to Turbo-Roundabouts. Procedia - Social and Behavioral Sciences, 111, 137-146.
Soleimani, A., & Nasrabadi, N. M. (2018). Convolutional Neural Networks for Aerial Multi-Label Pedestrian Detection. 2018 21st International Conference on Information Fusion (FUSION), 1005-1010.
Soviany, P., & Ionescu, R. (2018). Optimizing the Trade-off between Single-Stage and Two-Stage Object Detectors using Image Difficulty Prediction. In Proceedings of the 2018 20th International Symposium on Symbolic and Numeric Algorithms for Scientific Computing.
Sreedhar, K., & Panlal, B. (2012). Enhancement of images using morphological transformations. Int. J. Comput. Sci. Inf. Technol(4), 33–50.
Stuparu, D.-G., Ciobanu, R.-I., & Dobre, C. (2020). Vehicle Detection in Overhead Satellite Images Using a One-Stage Object Detection Model. Sensors, 20(22).
Systems, T.-T. S. (2011). Aimsun (Advanced Interactive Microscopic Simulator for Urban and Non-Urban Networks).
Tan, L., Huangfu, T., & Wu, L. (2021). Comparison of RetinaNet, SSD, and YOLO v3 for real-time pill identification. BMC Medical Informatics and Decision Making, 21.
Tang, T., Zhou, S., Deng, Z., Zou, H., & Lei, L. (2020). Vehicle detection in aerial images based on region convolutional neural networks and hard negative example mining. Sensors, 20(22).
The New York Times. (2021, 04 18). 2 Killed in Driverless Tesla Car Crash, Officials Say. Retrieved 12 31, 2021, from https://www.nytimes.com/2021/04/18/business/tesla-fatal-crash-texas.html
Tollazzi, T., Rencelj, M., & Turnsek, S. (2011). New Type of Roundabout: Roundabout with “Depressed” Lanes for Right Turning – “Flower Roundabout”. PROMET - Traffic&Transportation, 23.
Troutbeck, R. J. (1989). SR45 evaluating the performance of a roundabout.
Viola, P., & Jones, M. (2001). Rapid object detection using a boosted cascade of simple features. In Proceedings of the 2001 IEEE Computer Society Conference, 1.
Viola, P., & Jones, M. (2004). Robust real-time face detection. Int. J. Comput. Vis., 57, 137–154.
World Health Organization. (2021, 06 21). Road traffic injuries. Retrieved 12 31, 2021, from https://www.who.int/news-room/fact-sheets/detail/road-traffic-injuries
World Health Organization. (n.d.). Decade of Action for Road Safety 2011–2020. Retrieved 12 30, 2021, from https://www.who.int/publications/i/item/decade-of-action-for-road-safety-2011- 2020
Xie, Y., & Ji, Q. (2002). A new efficient ellipse detection method. Pattern Recognition, 2002. Proceedings. 16th International Conference on, 2.
Yap, Y. H., Gibson, H. M., & Waterson, B. J. (2013). An International Review of Roundabout Capacity Modelling. Transport Reviews, 33(5), 593-616.
Yap, Y. H., Gibson, H., & Waterson, B. (2015). Models of Roundabout Lane Capacity. Transportation engineering journal of ASCE, 141.
Yu, Y., Gu, T., Guan, H., Li, D., & Jin, S. (2019). Vehicle detection from high-resolution remote sensing imagery using convolutional capsule networks. IEEE Geoscience and Remote Sensing Letters, 16(12), 1894-1898.
Zhong, J., Lei, T., & Yao, G. (2017). Robust Vehicle Detection in Aerial Images Based on Cascaded Convolutional Neural Networks. Sensors, 17(12).
Zoph, B., Cubuk, E., Ghiasi, G., Lin, T.-Y., Shlens, J., & Le, Q. (2020). Learning Data Augmentation Strategies for Object Detection. Computer Vision - ECCV 2020, 566-583.
Zou, Z., Shi, Z., Guo, Y., & Ye, J. (2019). Object Detection in 20 Years: A Survey. arXiv:1905.05055v2.
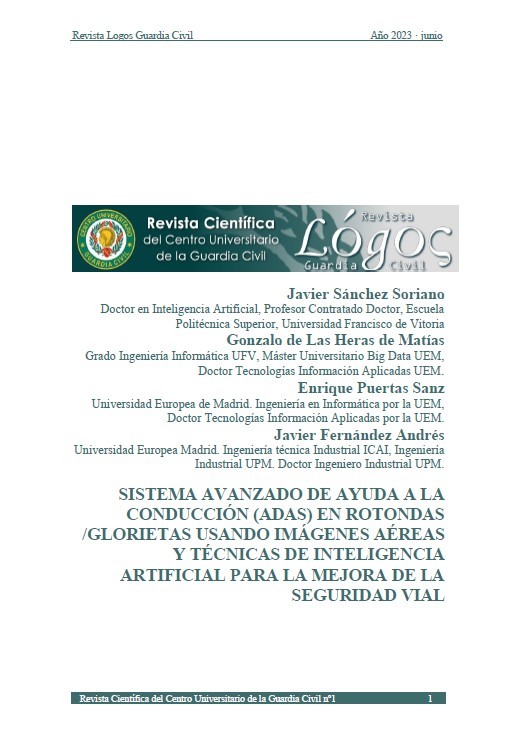
Downloads
Published
Versions
- 2023-06-30 (2)
- 2023-06-28 (1)
How to Cite
Issue
Section
License
Copyright (c) 2023 Javier Sánchez Soriano, Gonzalo De-Las-Heras, Enrique Puertas, Javier Fernández-Andrés

This work is licensed under a Creative Commons Attribution-NonCommercial-NoDerivatives 4.0 International License.
-
Attribution — You must give appropriate credit, provide a link to the license, and indicate if changes were made. You may do so in any reasonable manner, but not in any way that suggests the licensor endorses you or your use.
-
NonCommercial — You may not use the material for commercial purposes.
-
NoDerivatives — If you remix, transform, or build upon the material, you may not distribute the modified material.
- No additional restrictions — You may not apply legal terms or technological measures that legally restrict others from doing anything the license permits.